
Smart Microscopy
Smart Microscopy workflows use on-the-fly image analysis to continuously monitor the sample, give real-time feedback to the imaging software, and program the microscope to change its parameters during experimental acquisition - all this without human intervention.
Why using Smart Microscopy
Biological systems are inherently complex, and the response of even the more stable experimental model (e.g. cell line) can be highly heterogeneous. Therefore, many samples must be imaged to achieve statistically meaningful results. In microscopy, obtaining sufficiently large quantitative datasets during manual operation can become very time-consuming because the operator must first identify the targets of interest and then acquire each with experiment-specific settings. This workflow limits the number of events that can be recorded and the reproducibility of the results, especially when the phenotypes of interest are rare or occur only during specific biological stages.
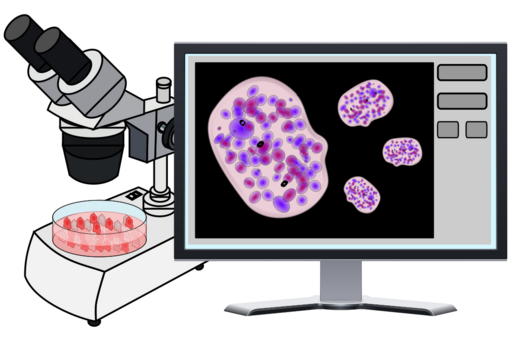
What is Smart Microscopy
To overcome the limitations of quantitative biology, much effort has been made over the last decade to connect bioimage analysis (used to, e.g., detect specific cells in microscopy images) with fully motorized and computer-controlled microscopes (via proprietary or open-source software packages) to generate automated and adaptive imaging workflows, referred to as smart microscopy. These automated workflows have also been used to handle complex changes in the sample's environment, in applications such as immunohistochemistry, sequential super-resolution microscopy, and fluorescence multiplexing imaging, by controlling complex liquid handling equipment together with adaptive feedback to the microscope. This feedback now allows researchers to, for example, follow imaging cues to trigger the fixation treatment when specific events, such as cell division, are observed.
Smart Microscopy Services in an Open-Access Facility
The interplay between image acquisition automation, image analysis, and Machine Learning is becoming a booming research field, allowing scientists to perform automated experiments that quantify large and complex data. The automation of end-to-end imaging workflows is not only an endeavour of academic groups, but also the microscopy industry at large is pushing toward microscopy automation, leveraging on image analysis and artificial intelligence. Smart microscopy will be an important trend in years to come; innovation will be stimulated by improvements and seamless integration of all the aspects listed above. Deep learning is likely to be pivotal in such improvements, as well as in strategies for handling the data deluge associated with such imaging. Therefore, the CCI is actively working on creating and implementing smart microscopy workflows at the infrastructure level as a service to our scientific community, making them available to any researcher thanks to the high technical and scientific skill of our staff.
Which microscopes are available for Smart Microscopy?
We are working on developments to make both light and electron microscopes compatible with Smart Microscopy workflows. However, at the moment this service is only available for:
- Celldiscoverer 7 with LSM900: The CD7 is a highly automated boxed light microscope, dedicated to live cell imaging, high content screening and other large experiments. It detects type of sample, adjusts the optics accordingly and autofocuses on the sample. It also contains the sensitive LSM900 Airyscan for high resolution imaging.
- AxioImager Z2: The upright AxioImager.Z2 from Carl Zeiss is a highly automatic widefield microscope, which is controlled by the Zen Blue software. It is generally used during CAT applications due to its flexible and heavy duty sample stage.
- AxioObserver Z1: The inverted Axio Observer. Z1 from Carl Zeiss is a highly automatic widefield microscope, which is controlled by the Zen Blue software. It is also equipped with the Apotome system for obtaining thinner optical sections than standard widefield microscopes.
Application example - Cellular 3D volume by Array Tomography
Many biological functions depend critically upon fine details of tissue’s molecular architecture that have resisted exploration by existing imaging techniques. Array tomography (AT) encompasses light and electron microscopy modalities that offer unparalleled opportunities to explore 3D cellular architectures of large samples in extremely fine structural and molecular detail (see figure). Fluorescence-AT achieves much higher resolution and molecular multiplexing than most other fluorescence microscopy methods, while electron-AT can capture 3D ultrastructure easily and rapidly compared to traditional serial-section electron microscopy methods. The Correlative mode of Array Tomography (CAT) furthermore offers the unique capacity of merging the molecular discrimination strengths of multichannel fluorescence microscopy with the ultrastructural imaging strengths of electron microscopy.
However, performing CAT has many challenges, including sample preparation, image acquisition and data handling. This approach results in imaging workflows which are notoriously time-consuming and require high levels of expertise. Furthermore, in CAT the microscopes have very different fields of view, and therefore accurately overlaying the different 3D volumes is highly non-trivial and prone to bias. In this framework, the CCI is working on smart microscopy workflows to increase the image acquisition speed of AT in both light and electron microscopy by implementing denoising methods, managing big image data, and automating some of the target identification steps that currently need human intervention.
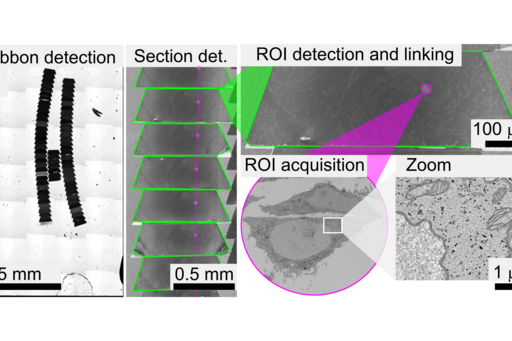
Interdisciplinary Method Development
To effectively implement smart microscopy solutions the interaction between microscopists, software developers, bioimage analysts, and end-users must be facilitated by a user-friendly IT infrastructure that allows collaborative workflows. To achieve this, we are building upon collaborative frameworks such as APEER (cloud-based platform for image processing workflows, and training of deep-learning models, Carl Zeiss, https://www.apeer.com), and Cytomine (web platform to organize, visualize, semantically annotate, and analyze image data, University of Liège, https://uliege.cytomine.org/). These tools facilitate the training of Deep Learning (DL) models by allowing users to collaboratively annotate image datasets, and then request the training and evaluation of DL models on dedicated image analysis servers.
Democratization of imaging resources
The collaborative web-based frameworks we are working on will promote the democratization of resources by decentralizing the access to powerful computational tools to teams in different locations.
Thanks to smart microscopy workflows as well as remote access to automated microscopy equipment, we can further allow access from external users to microscopy equipment at CCI. We are currently optimising shipping procedures of biological samples for imaging over long periods of time, and outside of office hours. Thus, external users get access to critical imaging infrastructure and expert knowledge, while the CCI gains important expertise by being exposed to a broader range of user projects.

Support by the SSF - Research Infrastructure Fellows 2
We are happy to announce that Rafael Camacho at CCI has been selected in 2022 as a Research Infrastructure Fellow by SSF (SEK 15 million during a five-year period). We are proud to be recognized by SSF for the second time, this is a truly remarkable achievement. Link to the SSF page
This SSF programme is intended to contribute to the development and support of research infrastructures, to increase national and international user-accessibility, as well as to enhance strategic career paths for specialists within academia.
Interested in our Smart Microscopy services?
If you are interested in Smart Microscopy Services, or in the development of these novel methods please contact us.
Further Reading
- Karras, C. et al. Optics Communications 436, 69–75 (2019). (External link)
- Banote, R. K. et al. Scientific Reports 10, 10127 (2020). (External link)
- Conrad, C. et al. Nature Methods 8, 246–249 (2011). (External link)
- Edelstein, A. et al. Current Protocols in Molecular Biology 92, (2010). (External link)
- Pinkard, H. et al. Nature Methods 13, 807–809 (2016). (External link)
- Pinkard, H. et al. Nature Methods 18, 226–228 (2021). (External link)
- Tosi, S. et al. Frontiers in Bioinformatics 1, (2021). (External link)
- Fuqua, T. et al. Scientific Reports 11, 10314 (2021). (External link)
- Booth, B. W. et al. iScience 2, 136–140 (2018). (External link)
- Almada, P. et al. Nature Communications 10, 1223 (2019). (External link)
- Moore, J. et al. Nature Methods 18, 1496–1498 (2021). (External link)
- Pietzsch, T., et al. Nature Methods 12, 481–483 (2015). (External link)
- Vergara, H. M. et al. Cell 184, 4819-4837.e22 (2021). (External link)
- Tischer, C. et al. Bioinformatics 37, 3079–3081 (2021). (External link)