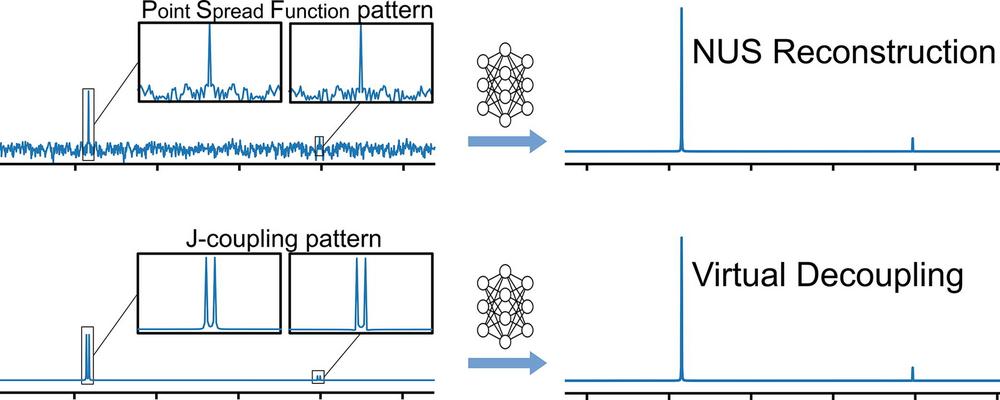
NMR and machine learning
Over the last recent years, AI and deep learning (DL) have led to impressive advances in many fields, including NMR spectroscopy.
The distinctive feature of DL neural networks (DNNs) is their ability to establish essential correlations between the input and output and thus to retrieve relevant multi-faceted prior information, e.g. about NMR signal, that is difficult to formulate in an analytical form and embed into a computer algorithm.
At the Swedish NMR Centre, we present a new deep neural network based on the WaveNet architecture (WNN), which is designed to grasp specific patterns in the 2D NMR spectra. In the context of non-uniform sampling (NUS) reconstruction, WNN trained at a fixed NUS schedule is the first tool that specifically uses a point spread function as prior knowledge in NUS spectra reconstruction. The pattern recognition by WNN is also demonstrated for successful virtual homo-decoupling in a 2D methyl-1H,13C-HMQC spectrum.