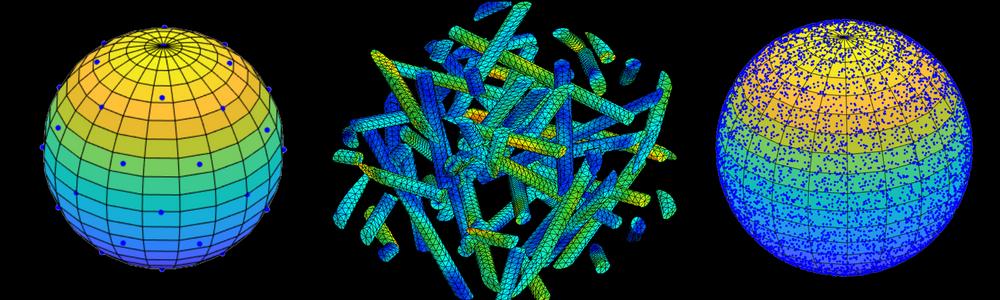
Mechanics and Physics of Materials
Short description
This group is primarily focused on mechanics and physics of micro-structurally heterogeneous materials. Micro-mechanical and coupled multi-scale models are developed to predict complex behavior of multi-phase materials, with an emphasis on (bio-)composites. To increase the predictive capability and to enhance the computational efficiency of the models, machine learning techniques, particularly artificial neural networks, are used. As an additional line of research activities, this group also works, in collaboration with other research groups, on bio-mechanics problems. Physics-based models are employed together with machine learning techniques to develop computationally enhanced bio-mechanical models.
News
Open PhD position
MSCA-DN project (DurAMat), “Deep-learning-enhanced multiscale modelling of moisture diffusion in bio-composites as coating for WAAM metal”. Application: https://duramat-project.eu/duramat/
PhD half-time seminar
Ehsan Ghane, “Multi-scale deep-learning for elastic and elasto-plastic behavior of woven composites”, June 16, 2023 (PJ Salen, Physics department) at 10:00.
Master thesis defense
Hon Lam Cheung, “Micromechanics-based artificial neural networks and transfer learning for modeling short fiber reinforced composites in automotive applications”, June 7, 2023 (von Bahr, Soliden, Physics department) at 14:00.
European grant application approved
April 2023: MSCA-DN application got approved. 10 PhD student positions will be defined within a consortium of European institutes and industries. 1 PhD student will join this group.
Projects
Micromechanics-based deep-learning modelling of short fiber composites
A wide variety of microstructural parameters, such as fiber volume fraction and fiber orientation distribution (among others) play an important role in the mechanical performance of short fiber reinforced composites. Thus, different homogenization schemes have been extensively investigated in different micromechanical models. However, due to different challenges such as expensive calculations and difficult generation of microstructural samples, it is needed to develop more sophisticated methods. In this project, we are using micro-mechanical simulations and artificial neural networks for developing data-driven models for complex behavior of these materials.
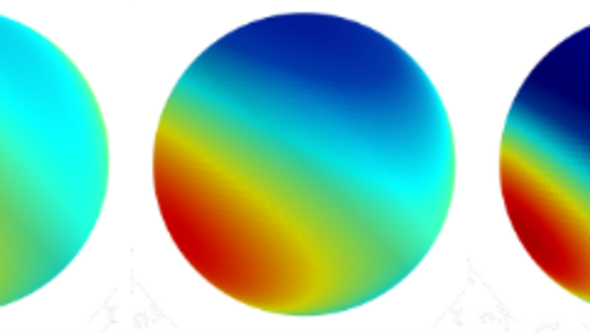
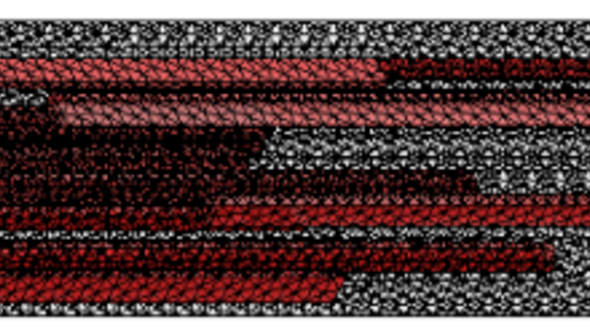
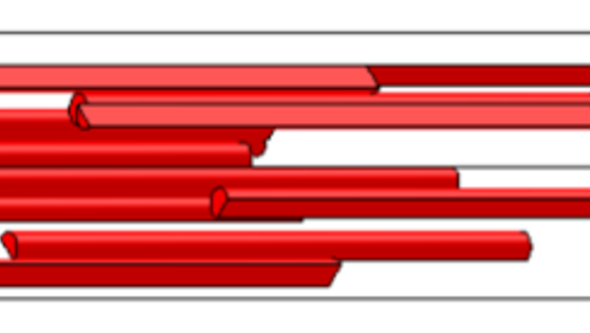
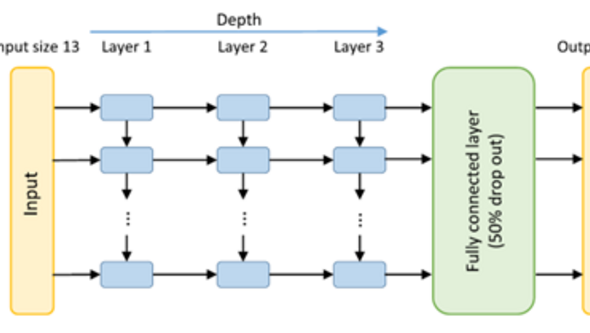
Related Publications
J. Friemann, B. Dashtbozorg, M. Fagerström, S.M. Mirkhalaf, (2023). A micromechanics-based recurrent neural networks model for path-dependent cyclic deformation of short fiber composites. International Journal of Numerical Methods in Engineering, 124:2292-2314.
N. Mentges, B. Dashtbozorg, S.M. Mirkhalaf, (2021). A micromechanics-based artificial neural networks model for elastic properties of short fiber composites. Composites Part B: Engineering, 108736.
Multi-scale artificial neural networks for modelling woven composites
Composite laminates, composed of layers of woven laminas, have advantages compared to laminates made from unidirectional laminas, specifically in design and manufacturing. These materials are obtaining an increasing number of applications in structural components, due to superior mechanical performance compared to unidirectional laminates and improved delamination resistance. However, modelling woven composites is very challenging due to (i) existing two heterogenous subscales, namely mesoscale and microscale, (ii) the interlaced nature of yarns, which results in developed complex stress states. In this project, we are developing deep-learning-enhanced multi-scale models for woven composites using mean-field and high-fidelity full-field simulations and artificial neural networks.
Related Publications
E. Ghane, M. Fagerström, S.M. Mirkhalaf, (2023). A multiscale deep learning model for elastic properties of woven composites. International Journal of Solids and Structures 282, 112452.
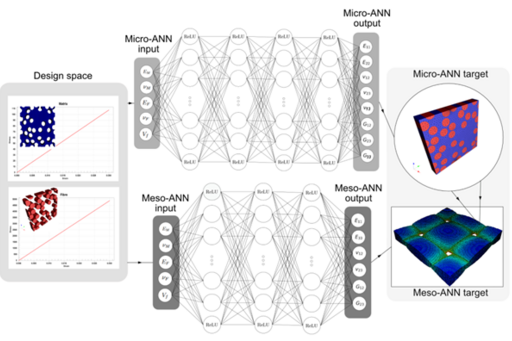