- Home
- News and events
- Find news
- Data is the key to understanding emissions of chemicals from Swedish households
Data is the key to understanding emissions of chemicals from Swedish households
In a FRAM Centre financed project, Mikael Gustavsson has estimated chemical emissions from Swedish households. He has also calculated the potential risks for the environment with the help of data and mathematical modelling. Now he presents his results.
Today’s consumer products include a lot of different chemicals. For example, textiles, pharmaceuticals, products for personal hygiene, cleaning or washing, stopping in furniture and paints all contain different chemicals, in varying amounts. When people use these products by cleaning their house, washing their clothes, or eating their medicines, some of the chemicals within the products are released into the wastewater system. When the water then passes through the wastewater treatment plant some of the chemicals are captured or degraded but some are released into lakes or the sea. But how much – and is it a problem?
Mikael Gustavsson works as a researcher at Mathematical Sciences, a department shared by Chalmers University of Technology and the University of Gothenburg. He is part of the FRAM team and his work is financed by the FRAM Centre for four years.
To find out how much chemicals that are emitted from Swedish consumers into the environment and how that amount connects with the risk of negative environmental impact – a lot of data from different sources needs to be processed. In a FRAM project, Mikael Gustavsson worked together with Sverker Molander, Thomas Backhaus, and Erik Kristiansson to try to find the answers.
The method
Mikael used a modelling pipeline to process the available data. The aim was to get better knowledge about Swedish households’ chemical emissions. This would in turn allow problematic consumer uses to be identified and then compared to other sources of environmental pollution, like agriculture or shipping. In the end, this would help guide chemical management and ensure that resources are spent where they have the biggest impact.
The results were presented in a FRAM Seminar. During the seminar, Mikael presented a modelling pipeline which includes a multi-step process.
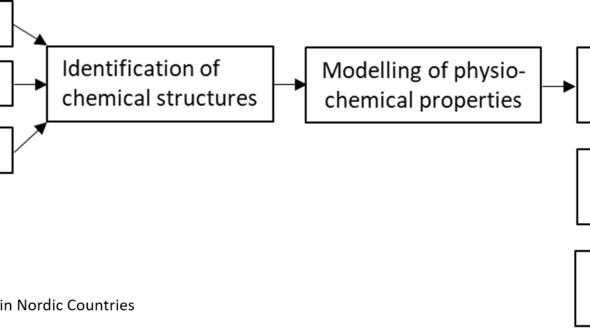
The modelling pipeline starts with gathering data for the three product-groups which are included in the study. The data is then processed in several steps, which includes clean-ups, additional data collection, inclusion of other modelling tools etc. before arriving at a final estimate of the risk in a typical Swedish wastewater treatment plant outflow.
How much chemicals are there?
In total, 3226 chemicals registered by the Chemical Abstracts Service (CAS) were included in the study. Out of these, 2007 were unique identified chemicals which the researchers could find a defined structure for.
To find out how much of these chemicals that are emitted into wastewater, Mikael had to determine both how much of the total use that was from a consumer setting and find information on how much of that use that would then end up in the waste water.
– The emission factor that we refer to is simply the percentage of a chemical compound that is used by a household that is then expected to end up in wastewater, Mikael explains.
So, what chemicals are emitted after the wastewater (sewage) treatment? After several modelling steps, Mikael’s calculations show that chemical substances used for cleaning, washing, and bleaching are the ones most emitted to the environment, together with complexing agents and chemicals from textiles.
Pharmaceuticals seem to be the most problematic products
The risk assessment of these chemicals is done with by dividing the outflow concentration from the wastewater treatment plant with an environmental threshold, i.e. a concentration which is considered to be safe in the environment. These results also show how much the outflow needs to be diluted for the chemical concentrations to be safe.
The results from the risk assessment changed the picture quite drastically. Even though chemical substances used for cleaning, washing, and bleaching are the ones most emitted to the environment, pharmaceuticals seem to have the highest risk for negative environmental impact. There is thus no correlation between the amount of chemicals that goes out into the environment and what risks they entail. However, it is no surprise for the researchers that pharmaceuticals seem to be the most problematic product group.
– No, it is not surprising, but as a modeller you get happy when the results end up as you guessed that they would, says Mikael.
Since the pharmaceuticals showed a higher risk level, they were analysed a bit deeper and it turned out that two substances drive the total risk.
– This is a pattern that we see very often. A few compounds drive the risks of the mixture. For example, when we work with real world samples from different locations, or different times, it is often different chemicals between the samples. But the pattern is almost always there.
Accuracy versus the unknown
After having presented all the steps in the method, Mikael came back to the number of chemicals that could be included in the study. From the beginning they were 3226 but only 2007 were unique identified chemicals with a known structure. Out of these, as much as 2/3 lacked a defined environmental threshold, i.e. a concentration predicted to have no effect. This means that there is not enough data available to determine the risk of these chemicals.
– The modelling can never be better than the available data. To get more accurate results, a lot more data is needed. We need to know more both about the consumer use and exposure, as well as get access to more hazard data. This is especially frustrating for pharmaceuticals where we know that a lot of data exist, but they are not publicly available.
To make the results from the whole process easy to grasp, Mikael put together a table which shows an estimation of the accuracy or availability of data for the various steps in the process.
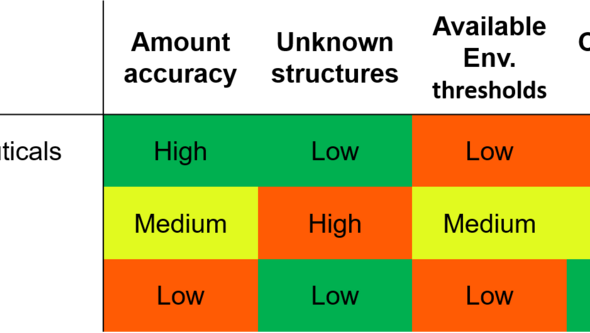
In the table you can see that, for example, the dataset for pharmaceuticals has a high amount of accuracy, a low amount of unknown structures while the availability of environmental threshold is low, and the calculated risk is high.
– We started off with three different data-sources, and we quickly noticed that each one of them came with their own challenges. These challenges are dependent on why the data was collected in the first place. Of course, none of these data-collections were originally designed to be used to estimate consumer emissions.
The results of the modelling confirm that it is possible to identify problematic compounds and uses by using this model framework. However, before we get the full picture a lot more knowledge needs to be generated.
The results in this article come from a FRAM Centre financed project including FRAM researchers Mikael Gustavsson, Sverker Molander, Thomas Backhaus and Erik Kristiansson. The results were presented at a FRAM Seminar held on the 25th of February.
Read more about the FRAM researchers on the FRAM website.
FRAM is a Centre for Future Chemical Risk Analysis and Management at the University of Gothenburg that works for a safe use of chemicals for both humans and the environment.
Read more about the FRAM Centre.