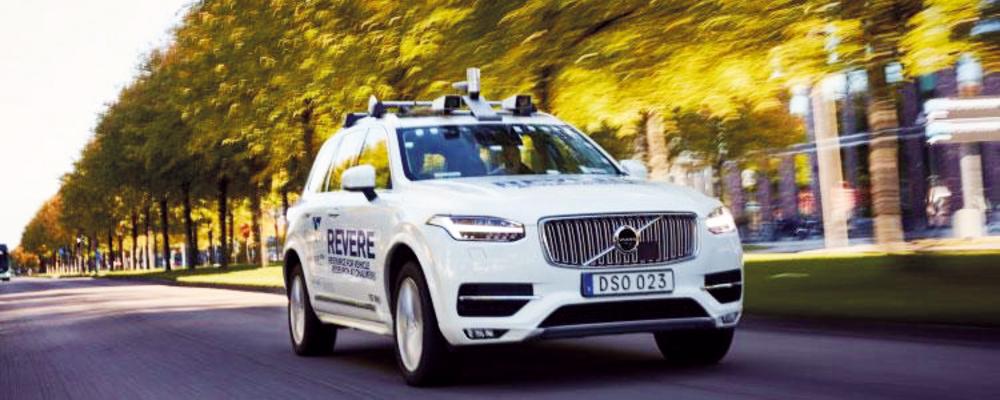
- The magic and complexity of self-driving cars
- Film to digitize the physical world
- Hard to judge when the AI system has the right amount of data
- Detailed mapping of the causal combination in case of a possible mistake
- A self-driving car requires a large collaboration between different competencies
- The films from the data collection often need to be saved some time for analysis
- Difficult to get around issues related to privacy during filming
- Incomplete representation is a challenge
- Assisted driving or a self-driving car?
Complex process when the next generation of self-driving cars are developed
At the tech company Asymptotic AI, a research project is underway for safer systems for self-driving cars and assistance systems. The project uses a car with six different cameras and also a laser scanner that measures the distance to objects. The car drives around in Gothenburg and collects data to be used to train the AI systems.
Yinan Yu is one of the co-founders of the company Asymptotic AI, but also an assistant professor at the Department of Computer and Information Technology.
– My doctorate is actually from the field of electrical engineering – more precisely signal processing and machine learning. The reason for entering the field of machine learning was my great interest in optimization. To optimize something with the help of a computer, you describe the goal you want to achieve in a way that a computer can understand, i.e. in a programming language. You give the computer all possible variables that you can influence, and the computer can tell you in an instant which solution is optimal for these particular variables, says Yinan Yu.
– To me the optimization process was magical, but at the same time very precise and rigorous. Machine learning is a further development of the field of optimization – with an increased element of magic if you put it that way. About five or six years ago, the automotive industry became one of the first areas with a lot of research and development in machine learning and AI.
– It is fantastic to see that the technology develops so quickly. There are so many people nowadays working with the combination AI and vehicles and the area is really moving forward. Self-driving cars and assistance systems is a super interesting subject area and there is so much to say, says Yinan Yu.
The magic and complexity of self-driving cars
– When we train our test car, the overall goal of the entire process is for the car to make the right decisions – decisions that a human can understand and agree with.
– Perception, i.e. handling and interpretation of information, is the basis for the entire system of self-driving cars. If you cannot perceive and analyze your surroundings, there is no chance that you will be able to make the right decision. For the perception part, you need two things: you need to see and you need to understand what you see. It applies to living beings and to machines that use artificial intelligence to perform something.
– The car must initially have knowledge of all traffic rules, "understand" what it "sees" in traffic and then make the right decision in an instant. It is a very complex process to achieve, but if the autonomous system is built the right way, you have the advantage that it is so much faster and also more predictable and consistent than a human. An AI system doesn't get sleepy, stressed and have no problems with concentration.
Film to digitize the physical world
– The filming that takes place when we drive around with the test car involves a data collection process where we digitize the physical world around us and save it in some kind of digital format. Almost all automated monitoring processes actually work that way. The datasets from the films are then used to train artificial intelligence and to evaluate our AI systems.
– The start-up company that I am one of the co-founders of works with what is called data-centric and human-centric AI. Data-centric AI means a focus on qualitative data. In the big-data era, it was a bit of "the more data the better", but now the trend is that we only want high-quality data. A very large amount of data is today seen more as a burden, you have to run the data on a large server for example, which means a lot of energy will be consumed and resources will be wasted, says Yinan Yu.
– Human-centered AI is about AI optimally serving people. Industrial Revolution 4.0 involved automation – Industrial Revolution 5.0 has a focus on people. From that perspective, the issue of surveillance is very interesting.
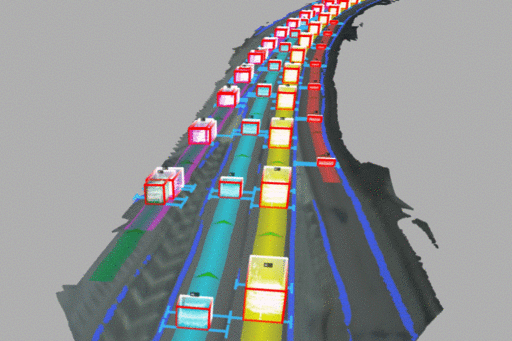
Hard to judge when the AI system has the right amount of data
When data has been collected via the test car for a longer period of time, the data iteration process starts. The researchers look at their collected data and consider whether is time to let the process be automated. Sometimes the developers discover gaps in the collected data, and might say something like: "ok we need some more recordings, we don't have enough rainy days and today it's raining so today's collected data will be very valuable".
– Each development cycle for the AI system is quite long. You have your goals set and you have all the variables that must be included, but what we want the car to achieve is still too complex for it to be possible to search for the optimal combination of all variables at the same time. In other words, it is very difficult to determine when you have the right amount of data for the car's mission. Usually, the researchers can still reason their way to that. Here, experience and intuition enter the process, combined with specifications and requirements from other development teams, making the dynamics very complex. The development of the AI system needs to be evaluated at regular intervals and the system needs, for example, to meet the requirements of all traffic safety regulations, says Yinan Yu.
Detailed mapping of the causal combination in case of a possible mistake
– If your test car makes a mistake, of course you need to find out why. Is it from a machine learning perspective – maybe the AI model doesn't have enough data for the area or the scenario? Or is it maybe a more car-related problem, something to do with the mechanics? A mistake usually requires a large amount of analysis to find the correct causal relationship. An accident database can also be used to try to understand the scenario.
– Trying to understand a problem that has arisen where AI is included as a component is anything but trivial, says Yinan Yu. "Deep learning" which is what AI is about is a bit like a black magic box. AI creates magic, but even for a scientist it can be hard to understand why. AI as a technology is very much about compressing very large data sets and if something is missing from the data set, the AI will simply not be able to make the right judgements.
– Explainable AI is therefore an important research direction. How can we ensure that the AI system will continue to learn continuously the more valuable data we collect? It is still an open research question and a very active and important field of research. You don't want a to create a system that can't continue to evolve, says Yinan Yu.
A self-driving car requires a large collaboration between different competencies
The security experts involved in the development process are of course not the same people as the AI experts in the team. The process of developing a self-driving car is a team effort that is more difficult than people might think.
– Car companies often seem to think "We take excellence from the AI field and combine it with excellence in safety and we will then create something absolutely fantastic". But sometimes you get only half the effectiveness from each group, as they need to navigate because they speak completely different languages. This can be a big challenge from a social point of view. Every car company takes the latest in AI and the latest in safety and then it's up to you as a researcher to make the groups work together – and also conduct your own research – to make all of this into a coherent system.
The films from the data collection often need to be saved some time for analysis
– The usual thing when you have a self-driving vehicle filming the surroundings is that you need to save the recordings to use them for development purposes. The analysis of the data is crucial for the quality of the systems being built and very important for the safety. But larger volumes of data collection and further AI development are still needed before self-driving vehicles can be used more widely, says Yinan Yu.
– There are many scenarios that can become very critical in the automotive industry, where you have no room to allow yourself to fail. That's why self-driving vehicles haven't been put on the roads yet, we don't really have control over them.
– You train your AI model for a long time before putting it into a car to test the system in traffic. The researchers start the process by doing a very large number of simulations in their computers. At some point, they decide the results are good enough to test the model in a car. In order to be allowed to do tests in traffic, various certificates are also required first, so it is clear who is responsible for the car and its actions.
Difficult to get around issues related to privacy during filming
– GDPR is something that must be taken into account when we train our research vehicle. Filming and recording people in the street is clearly an invasion of privacy, but since we drive around with cameras on the car, filming people cannot be avoided. One solution could be to have very visible information on the car that you are being filmed when the car passes by, says Yinan Yu.
– We have also constructed anonymization software that blurs both people's faces and the number plates caught on film. But it is not always enough to blur people's faces, it is still possible to recognize them based on their body shape, clothes and other things. There are several other anonymization programs that Asymptotic AI uses in its research and product development. For example, the programs can erase an entire body, and according to Yinan Yu, the development of new versions of these programs is a necessary step.
It is still not fully mapped out what are the possible consequences of collecting, analyzing and saving film recordings of people out on the streets. The legislation surrounding this type of recording is not yet fully developed, leaving room for uncertainty.
Incomplete representation is a challenge
Another challenge that Yinan Yu sees is that AI easily can create a skewed representation into the data used.
– If there are a lot of young people in the streets in your training data, for example, and the AI system has learned to understand how young people move, the system will distinguish that particular category better. During a training round withed the car, an elderly person will probably not be identified as easily by the system, because elderly people move slowly and so on. A biased data collection can apparently become very dangerous...
– The problem of built-in bias in data sets is something I would really like to work on further. Problems can arise if the needs of all groups not are taken into account when building a safe city and vehicle assistance system, says Yinan Yu.
Assisted driving or a self-driving car?
Some developers invest in fully autonomous driving, where the car itself has to make the decisions in traffic. Others believe more in assisted driving, where the car helps the driver with things like auto braking and similar functions.
– Personally, I perhaps believe more in self-driving public transport than in a scenario where everyone drives around in a self-driving car – that's too much of a luxury, haha! We also need to think about sustainability and the environment.
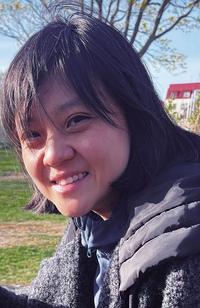
Yinan Yu
Assistant professor
Division of Computing Science
Department of Computer Science and Engineering